Trading strategy
Most of the scientific literature credits algorithmic trading with beneficial effects on market quality, liquidity, and transaction costs. Only a few papers highlight possible risks imposed by the greatly increased trading speed. However, all academics encourage objective assessments as well as sound regulation in order to prevent system failures without cutting technological innovation. This section concentrates on major findings regarding the U. Impact, on trade modification and cancellation rates, market liquidity, and market volatility.
Oxford Algorithmic Trading Programme | Saïd Business School
Among the first who analyzed algorithmic trading pattern in electronic order books, Prix et al. Owing to the characteristics of their data set, they are able to identify each order by a unique identifier and so re create the whole history of events for each order. As they focus on the lifetimes of the so-called no-fill deletion orders, that is, orders that are inserted and subsequently cancelled without being executed, they find algorithm-specific characteristics concerning the insertion limit of an order compared to ordinary trading by humans.
Gsell and Gomber likewise focus on differences in trading pattern between human and computer-based traders. In their data setup they are able to distinguish between algorithmic and human order submissions. They conclude that automated systems tend to submit more, but significantly smaller, orders. Additionally, they show the ability of algorithms to monitor their orders and modify them so as to be at the top of the order book. The authors state that algorithmic trading behavior is fundamentally different from human trading concerning the use of order types, the positioning of order limits, modification or deletion behavior.
Algorithmic trading systems capitalize on their ability to process high-speed data feeds and react instantaneously to market movements by submitting corresponding orders or modifying existing ones. Algorithmic trading has resulted in faster trading and more precise trading strategy design, but what is the impact on market liquidity and market volatility?
The following sections provide a broader insight to this question. Harris , p. Hendershott et al. This event marked the introduction of an automated quoting update, which provided information faster and caused an exogenous increase in algorithmic trading and, on the other side, nearly no advantage for human traders. By analyzing trading before and after this event, the authors find that algorithmic trading lowers the costs of trading and increases the informativeness of quotes.
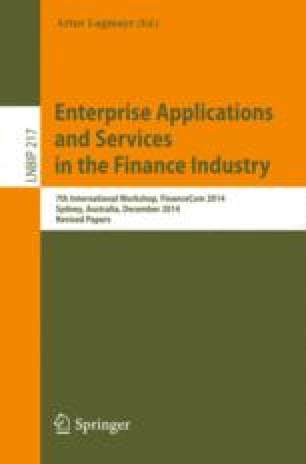
Hendershott and Riordan confirm the positive effect of algorithmic trading on market quality. They find that algorithmic traders consume liquidity when it is cheap and provide liquidity when it is expensive. Further, they conclude that algorithmic trading contributes to volatility dampening in turbulent market phases because algorithmic traders do not retreat from or attenuate trading during these times and therefore contribute more to the discovery of the efficient price than human trading does.
These results are backed by findings of Chaboud et al. Based on a data set of algorithmic trades from to , the authors argue that computers provide liquidity during periods of market stress. Overall these results illustrate that algorithmic trading closely monitors the market in terms of liquidity and information and react quickly to changes in market conditions, thus providing liquidity in tight market situations Chaboud et al. Among the theoretical evidence on the benefits of algorithmic trading, the model presented by Foucault et al.
In order to determine the benefits and costs of monitoring activities of securities markets, the authors develop a model of trading with imperfect monitoring to study this trade-off and its impact on the trading rate. In order to study the effect of algorithmic trading, the authors interpret it as a reduction of monitoring costs, concluding that algorithmic trading should lead to a sharp increase in the trading rate.
Last, algorithmic trading is socially beneficial because it increases the rate at which gains from trades are realized. Yet adjustments in trading fees redistribute the social gain of algorithmic trading between participants. With research, however, this prejudice proves to be unsustainable. By simulating market situations with and without the participation of algorithmic trading, Gsell finds decreasing price variability when computers act in the market. This might be explained by the fact that because there is lower latency in algorithmic trading, more orders can be submitted to the market and therefore the size of the sliced orders decreases.
Fewer partial executions will occur because there will more often be sufficient volume in the order book to completely execute the small order. If fewer partial executions occur, price movements will be narrowed as the order executes at fewer limits in the order book. Assessing the foreign exchange market and basing their work on a data set that differentiates between computer and human trades, Chaboud et al. The authors use an ordinary least-squares approach in order to test for a causal relation between the fractions of daily algorithmic trading and to the overall daily volume.
Additionally, Groth confirms this relation between volatility and algorithmic trading by analyzing data containing a specific flag provided by the respective market operator that allows one to distinguish between algorithmic and human traders. The author indicates that the participation of algorithmic traders is associated not with higher levels of volatility, but with more stable prices.
Furthermore, algorithmic traders do not withdraw liquidity during periods of high volatility, and traders do not seem to adjust their order cancellation behavior to volatility levels. In other words, algorithmic traders provide liquidity even if markets become turbulent; therefore, algorithms dampen price fluctuations and contribute to the robustness of markets in times of stress. A more critical view of algorithmic trading is provided by researchers from the London-based Foresight Project.
Although they highlight its beneficial effects on market stability, the authors warn that possible self-reinforcing feedback loops within well-intentionedmanagement and control processes can amplify internal risks and lead to undesired interactions and outcomes Foresight The authors illustrate possible liquidity or price shock cascades, which also intensified the U.
Flash Crash of May 6, This hypothesis is backed, in part, by Zhang and Kirilenko et al. With increasing trading volume and public discussion, algorithmic trading became a key topic for regulatory bodies. After crises including the collapse of the investment bank Lehman Brothers and the Flash Crash, the regulators started probing and calling the overall automation of trading into question. Discussion is still intense, with supporters highlighting the beneficial effects for market quality and adversaries alert to the increasing degree of computer-based decision making and decreasing options for human intervention as trading speed increases further.
In the following we focus on a specific event that promoted regulators on both sides of the Atlantic to re-evaluate the contribution of algorithmic trading, the Flash Crash, when a single improperly programmed algorithm led to a serious plunge. We then present mechanisms currently in place to manage and master such events. On May 6, , U. Within several minutes equity indices, exchange-traded funds, and futures contracts significantly declined e. The CFTC together with the SEC investigated the problem and provided evidence in late that a single erroneous algorithm had initiated the crash.
This selling volume cascade flushed the market, resulting in massive order book imbalances with subsequent price drops. Intermarket linkages transferred these order book imbalances across major broad-based U. Finally, the extreme price movements triggered a trading safeguard on the Chicago Mercantile Exchange that stopped trading for several minutes and allowed prices to stabilize Commodity Futures Trading Commission b. In order to get a more detailed p. In Europe, a more flexible best-execution regime without re-routing obligations and a share-by-share volatility safeguard regime that have existed for more than two decades have largely prevented comparable problems Gomber et al.
Automated safeguard mechanisms are implemented in major exchanges in order to ensure safe, fair, and orderly trading. In the SEC implemented a marketwide circuit breaker in the aftermath of the crash of Octobe r 19, Black Monday. Based on a three-level threshold, markets halt trading if the Dow Jones Industrial Average drops more than 10 percent within a predefined time period NYSE In addition, many U.
So far, the academic literature provides mixed reviews regarding the efficiency of circuit breakers. Most of the studies conclude that circuit breakers are not helping decrease volatility Kim and Yang Chen finds no support for the hypothesis that circuit breakers help the market calm down. Nevertheless, the importance of such automated safeguards has risen in the eyes of regulators on both side of the Atlantic. On October 20, , the European Commission published proposals concerning the review of the MiFID framework and now requires trading venues to be able to temporarily halt trading if there is any significant price movement on its own market or a related market during a short period European Commission The demand for automation was initially driven by the desire for cost reduction and the need to adapt to a rapidly changing market environment characterized by fragmentation of order flow.
Algorithmic trading as well as HFT enable sophisticated buy side and sell side participants to achieve legitimate rewards on their investments in technology, infrastructure, and know-how. To draw a picture of the future evolution of algorithmic trading, it seems reasonable that even if the chase for speed is theoretically limited to the speed of light, the continuing alteration of the international securities markets as well as the omnipresent desire to cut costs may fuel the need for algorithmic innovations.
This will allow algorithmic strategies to further claim significant shares of trading volume.
- The Daily Routine of a Swing Trader!
- Mutual Funds and Mutual Fund Investing - Fidelity Investments.
- Momentum trading strategies;
- How the News Affects Stock Prices?
- ea trading systems;
- Momentum trading strategies.
- Main navigation.
Considering further possible shifts to the securities trading value chain, p. So far, the academic literature draws a largely positive picture of this evolution. Algorithmic trading contributes to market efficiency and liquidity, although the effects on market volatility are still opaque.
Therefore, it is central to enable algorithmic trading and HFT to unfold their benefits in times of quiet trading and to have mechanisms like circuit breakers in place to control potential errors at both the level of the users of algorithms and at the market level. Yet preventing use of these strategies by inadequate regulation resulting in excessive burdens may result in unforeseen negative effects on market efficiency and quality.
A Community For Your Financial Well-Being
Aite Group Algorithmic trading More bells and whistles. Algorithmic trading in FX: Ready for takeoff? Aldridge, I. High-Frequency Trading. Find this resource:. Bildik, R. Are price limits effective? Evidence from the Istanbul Stock Exchange. Journal of Financial Research 29 3 , — Chaboud, A. Chiquoine, E. Hjalmarsson, and C. Vega Rise of the machines: Algorithmic trading in the foreign exchange market.
Chaovalit, P. Zhou Ontology-supported polarity mining. Chen, Y. Price limits and stock market volatility in taiwan. Pacific-Basin Finance Journal 1 2 , — CME Group Algorithmic trading and market dynamics.